Your message was sent!
Share via:
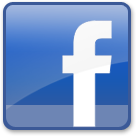
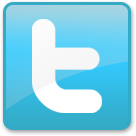
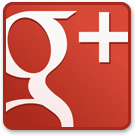
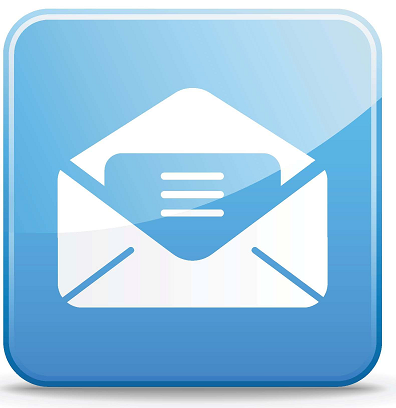
Share via e-mail:
From: | |
To: | |
Custom Message (optional): | |
Share on Facebook:
You must be logged into your Facebook account in order to share via Facebook.
Share via GooglePlus:
Click the button below to share this on Google+. A new window will open.
Share via Twitter:
You must be logged in to your Twitter account in order to share. Click the button below to login (a new window will open.)

Your message was sent!
Please log-in to your MaplePrimes account.
Wrong Email/Password. Please try again.
Automatically sign in on future visits |
Save this setting as your default sorting preference? |
|
Note: You can change your preference any time in your account settings | |
Don't show this again |
Please log-in to your MaplePrimes account.
Wrong Email/Password. Please try again.
Automatically sign in on future visits |